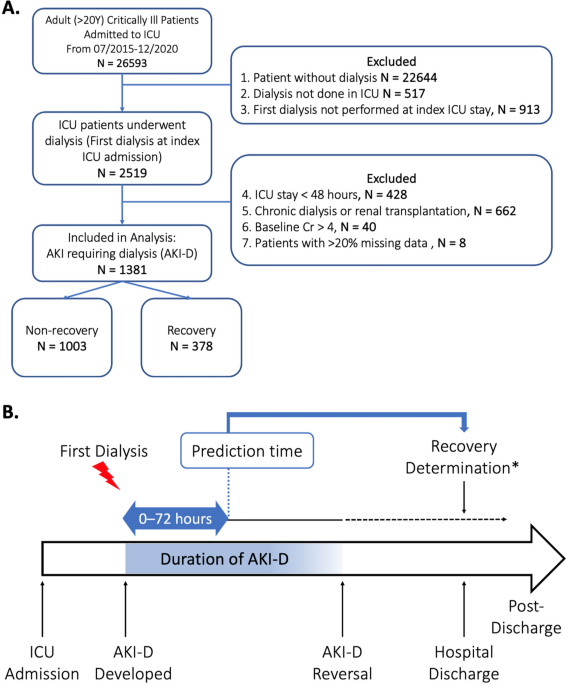
We constructed a machine-learning algorithm to forecast the early likelihood of dialysis liberation in critically ill patients with AKI, incorporating both the variability and trends of dynamic parameters from routine data within the first 72 h of dialysis. These models were developed, cross-validated, and temporal tested, thus demonstrating good discrimination in predicting renal recovery at hospital discharge. Furthermore, we applied class weighting to address data imbalance, used LASSO to develop models with few variables, and predicted short time windows (the first 24 or 48 h), all showing good discrimination. These results suggest the potential clinical utility of integration into EMR for clinical decision-support systems. Finally, using SHAP value and PDP, we identified critical features influencing the predictions of the model, with early vital signs and inputs/outputs domains being the vital drivers of the model. Explainable machine learning-based prediction for AKI-D recovery using existing EMR data hold potential for improving risk stratification and gaining insights into patient outcomes.
Nonrecovery of renal function after AKI-D is associated with increased morbidity and mortality and high health care cost25,26. Consistent with the findings of previous epidemiology studies1,26, the present study showed higher short-term (3-month) and long-term (1-year) post-discharge mortality rates for patients dependent on dialysis compared to those liberated from acute dialysis (Supplemental Figure S3). Early prediction of recovery from AKI-D in critically ill patients has significant implications regarding patient-centered care27. Currently, the prediction solely relies on clinical experience. The most commonly used dialysis cessation indicator is the increase in urinary output5. However, urinary output’s accuracy in predicting successful RRT discontinuation remains controversial, with reported AUROCs ranging from 0.63 to 0.91 and varying cut-off values5,28. Additionally, urinary output is typically employed as an indicator of renal recovery later upon RRT discontinuation rather than a marker in the early stages. Traditional functional biomarkers (serum/urine Cr or cystatin C) and novel biomarkers (kidney injury molecule-1, neutrophil gelatinase-associated lipocalin, osteopontin, tissue inhibitor of metalloprotease-2/insulin-like growth factor binding protein-7, proenkephalin A 119–159, etc.) have been explored as predictors of AKI-D recovery5,8,11,27,29. Current biomarkers for renal function recovery after AKI-D, which require additional samples and have limited conclusive evidence, have not been used widely to identify patients with a high probability of renal recovery in the early stages. The urgent need for precision guide to liberate from RRT was also recognized in the recent Acute Dialysis Quality Initiative (ADQI) consensus conference30. The experts emphasized on the integration of big data analysis and single case EMR evaluation to allow personalized RRT for every single individual. To address this gap, there is a clinical unmet need to integrate EMR to assess their predictive value for RRT discontinuation and prognosis in AKI-D.
Machine-learning models developed in critical nephrology can harness the data collected in EMR for important renal outcome predictions13,31,32. As data accumulates, these models will also offer the additional advantage of early prediction or enhanced accuracy. However, validated machine-learning models for predicting acute dialysis discontinuation in critical setting are less studied. To our knowledge, one prior research has employed a machine-learning approach to predict freedom from RRT in patients with AKI-D. Pattharanitima et al. utilized the Medical Information Mart for Intensive Care (MIMIC-III) database to predict RRT-free survival in critically ill patients with AKI requiring continuous renal replacement therapy (CRRT)16. Out of 684 patients, 30% had stopped from RRT successfully. Models using 81 features extracted between hospital admission and CRRT initiation yielded AUROC values ranging 0.43–0.7. In our study including 1,381 AKI-D individuals, we used 90 variables from the initial three days post-dialysis, including all vital signs and input–output records. Thus, variability and trends across multiple time points of these data were incorporated into our models. The prediction models in our study exhibited good performance, with AUROC of 0.77–0.81 in the development cohort and 0.82–0.85 in the temporal testing cohort. Aside from candidate predictors, the differences in model performance between our study and the prior study may also be due to differences in the study populations character, number of participants, and different feature window. The first three days are considered as acute phase of ICU patients, as exemplified by septic shock, where shock reversal often occurs within the first 3 days33. Importantly, providing additional prognostic information after initial intensive treatment period can aid in subsequent medical decisions, including the consideration of clinical trials for high-risk groups, or the potential withdrawal of life-sustaining medical care. Moreover, we trained models at 24 and 48 h in addition to the 72 h model, both maintaining good predictive performance.
As shown in Table 3 and Table S6, a proposed threshold of 0.5 for predicting renal recovery provided good specificity, whereas a threshold of 0.3 enhanced sensitivity. Decision curve analysis revealed the net benefits of using these models in clinical decision-making by considering the trade-offs between sensitivity and specificity at various threshold probabilities. The model’s use would yield more benefit than harm at both threshold of 0.5 and 0.3. Consequently, a lower threshold, such as 0.3, allowed for the identification of a broader subset of patients likely to recover renal function. Meanwhile, a threshold of 0.5 resulted in fewer false positives and would reduce alarm fatigue, a major concern in ICU alarm systems34,35. Therefore, the selection of a threshold in practical applications should be based on whether a healthcare provider requires assistance in accurately identifying patients who can recover or cannot recover after AKI-D, while effectively managing resources.
Using the interpretable machine-learning algorithm, nonsurprisingly, the single most influential variables in renal function recovery after initiating dialysis for AKI was urine output. Patients who successfully liberated from RRT demonstrated significantly higher urine output. According to PDP (Fig. 4), patients with urine volume > 1570 ml over the 72 h period post-dialysis were more likely to achieve dialysis independence at discharge. Figure 4 demonstrates the PDP of top predictors by SHAP value and the cut-off value in favor of renal recovery. In our study, the top 20 variables include previously well-studies factors for renal recovery, including urine volume or BUN5,36,37, along with less-explored variables (ex: enteral diet intake within the first 3 days after dialysis). Moreover, we categorized the top 20 variables identified by the XGBoost model by clinical domains, including comorbidity, vital signs, laboratory data, Inputs/outputs domain, and others. Besides urine volume, most of the early predictors were related to the vital signs domain (Fig. 3B). A general consensus exists that hemodynamic instability caused by excessive fluid removal during dialysis hinders renal recovery38. However, traditional prediction models for renal recovery have often overlooked vital signs due to their complexity and dynamic nature. Bellomo et al. conducted a retrospective study of critically ill patients with shock and found that higher levels of relative hypotension during the first few hours of vasopressor support were significantly associated with an increased risk of adverse kidney-related outcomes39. In line with the current evidence, our data suggests that early vital signs, not only the variance of systolic BP, but also SpO2, trend of respiratory rate, were significantly associated with the renal prognosis of critically ill patients. Additionally, the use of LASSO model with more limited variables and the incorporation of routinely collected laboratory data offer a practical means of rapid integration into EMR (Supplemental Table S8). An illustrate the interpretability of the models and the evolving of the key features over time using two separate individuals is presented in Supplemental Figure S4. Altogether, explainable machine-learning models can be deconvoluted to unveil new insights of how ICU patient features at the early stage interact with patient future events.
Strengths of our study include its size, comprising 1,381 patients with AKI-D among 26,593 ICU admissions. We also have complete data on vital signs and inputs/outputs with very low missing rates (< 1%). Furthermore, we linked the NHIRD cause-of-death data to mitigate withdrawal bias risk. This is especially important in ICU studies, as 40%–60% of critically ill patients with AKI-D have their treatment discontinued due to life support withdrawal or death4.
Our study has several limitations. First, recovery status was determined at hospital discharge; however, we recognized that dialysis liberation may proceed further. Nevertheless, the median hospital stay of critically ill patients with AKI-D was long (25.0 days for the entire cohort and 30.5 days for the recovery group), with dialysis-dependent catastrophic illness certificates verified before discharge by nephrologist among AKI-D nonrecovers. Thus, the kidney prognosis is clinically relevant. Second, our models made one-time early predictions of renal recovery on the basis of data obtained within 3 days of dialysis initiation. Events after that may drive the patient outcome away from the predictions. Though, we developed additional models at various time horizons (1 and 2 days), a continuously updating prediction is more appropriate for such cases. Third, limitations of our retrospective database include lack of other predictors of interest, including the degree of urine proteinuria, timed creatinine clearance, and novel kidney biomarkers, which may influence renal and patient recovery. Last, we used temporal testing, which is considered as an in-between validation of internal and external validation40. Although the recovery and mortality rates in this cohort was comparable to those reported in the literature4,26, the results should be further validated in other settings.
- The Renal Warrior Project. Join Now
- Source: https://www.nature.com/articles/s41598-024-63992-y