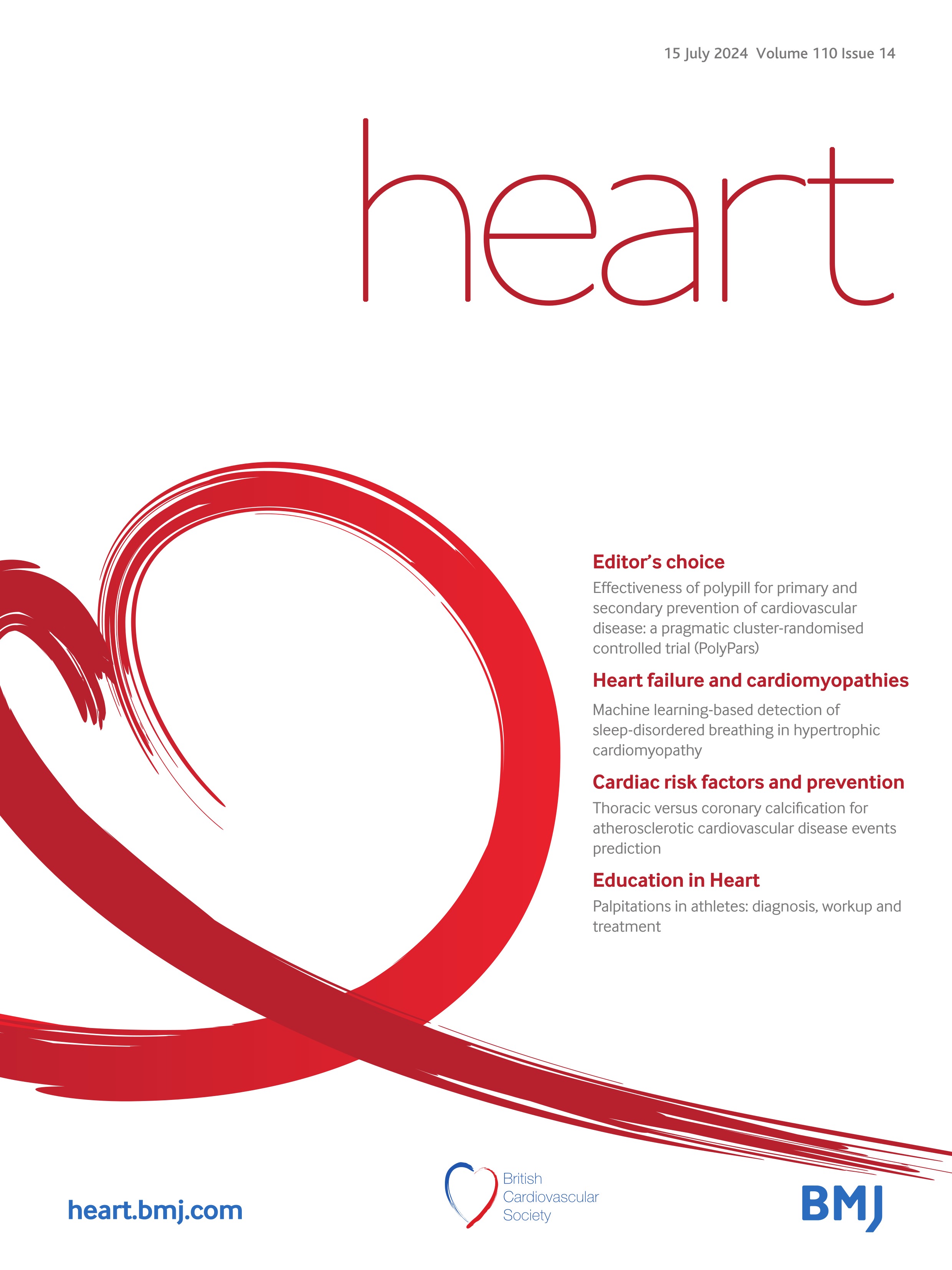
Hypertrophic cardiomyopathy (HCM) stands as one of the most common inheritable myocardial disease and is often marked by its association with sudden cardiac death (SCD) in the young.1 2 Traditionally, risk assessment in HCM has focused mainly on estimating the likelihood of SCD. However, with an increasing number of HCM diagnoses occurring in older individuals, there is an expanding emphasis on other significant cardiovascular complications, including heart failure, stroke and atrial fibrillation.3 This shift acknowledges that several clinical conditions contribute to cardiovascular outcomes in HCM, yet many risk factors remain to be discovered. Sleep-disordered breathing (SDB), including obstructive sleep apnoea (OSA), though not widely recognised by cardiologists, is highly prevalent in the general population and associated with various adverse cardiovascular outcomes in HCM.4 Timely interventions such as continuous positive airway pressure (CPAP) therapy can prevent adverse cardiac remodelling and potentially enhance long-term prognosis, underscoring the importance of early diagnosis. However, when patients with HCM are referred to cardiologists, SDB, a significant and common risk factor for cardiovascular outcomes, may not be promptly identified due to unfamiliarity.
Machine learning (ML)-based models, incorporating multidimensional variables, have demonstrated the ability to predict adverse events with greater precision and potential generalisability than traditional risk predictors across various cardiovascular diseases. These models play a crucial role in identifying important, …
- The Renal Warrior Project. Join Now
- Source: http://heart.bmj.com/cgi/content/short/110/14/931?rss=1