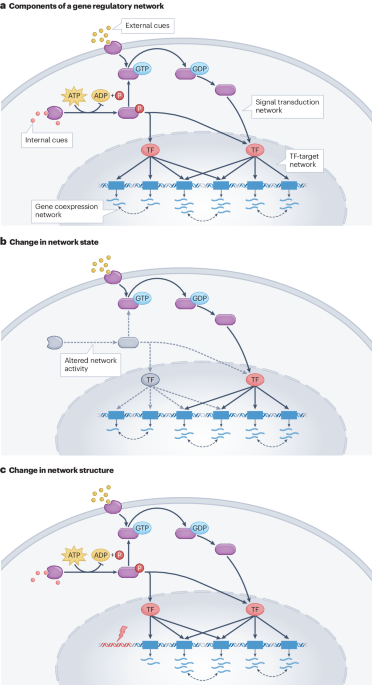
Enge, M. et al. Single-cell analysis of human pancreas reveals transcriptional signatures of aging and somatic mutation patterns. Cell 171, 321–330.e14 (2017).
Martinez-Jimenez, C. P. et al. Aging increases cell-to-cell transcriptional variability upon immune stimulation. Science 355, 1433–1436 (2017).
Maurano, M. T. et al. Systematic localization of common disease-associated variation in regulatory DNA. Science 337, 1190–1195 (2012).
Lee, T. I. & Young, R. A. Transcriptional regulation and its misregulation in disease. Cell 152, 1237–1251 (2013).
Southworth, L. K., Owen, A. B. & Kim, S. K. Aging mice show a decreasing correlation of gene expression within genetic modules. PLoS Genet. 5, e1000776 (2009).
Yang, J.-H. et al. Loss of epigenetic information as a cause of mammalian aging. Cell 186, 305–326.e27 (2023).
Levy, O. et al. Age-related loss of gene-to-gene transcriptional coordination among single cells. Nat. Metab. 2, 1305–1315 (2020).
Tatar, M. et al. A mutant Drosophila insulin receptor homolog that extends life-span and impairs neuroendocrine function. Science 292, 107–110 (2001).
Blüher, M., Kahn, B. B. & Kahn, C. R. Extended longevity in mice lacking the insulin receptor in adipose tissue. Science 299, 572–574 (2003).
Kamimoto, K. et al. Dissecting cell identity via network inference and in silico gene perturbation. Nature 614, 742–751 (2023).
Levin, M. Bioelectric signaling: reprogrammable circuits underlying embryogenesis, regeneration, and cancer. Cell 184, 1971–1989 (2021).
Kann, M. et al. Genome-wide analysis of Wilms’ tumor 1-controlled gene expression in podocytes reveals key regulatory mechanisms. J. Am. Soc. Nephrol. 26, 2097–2104 (2015).
Talyan, S. et al. CALINCA — a novel pipeline for the identification of lncRNAs in podocyte disease. Cells 10, 692 (2021).
Wang, Y. et al. Global transcriptomic changes occur in aged mouse podocytes. Kidney Int. 98, 1160–1173 (2020).
Ettou, S. et al. Epigenetic transcriptional reprogramming by WT1 mediates a repair response during podocyte injury. Sci. Adv. 6, eabb5460 (2020).
Guo, J.-K. et al. WT1 is a key regulator of podocyte function: reduced expression levels cause crescentic glomerulonephritis and mesangial sclerosis. Hum. Mol. Genet. 11, 651–659 (2002).
Palmer, A. K. et al. Targeting senescent cells alleviates obesity-induced metabolic dysfunction. Aging Cell 18, e12950 (2019).
Shankland, S. J. et al. Podocyte aging: why and how getting old matters. J. Am. Soc. Nephrol. 32, 2697–2713 (2021).
Aibar, S. et al. SCENIC: single-cell regulatory network inference and clustering. Nat. Methods 14, 1083–1086 (2017).
Singh, A. et al. Hippo signaling mediators Yap and Taz are required in the epicardium for coronary vasculature development. Cell Rep. 15, 1384–1393 (2016).
Kim, S. I. et al. TGF-β-activated kinase 1 is crucial in podocyte differentiation and glomerular capillary formation. J. Am. Soc. Nephrol. 25, 1966–1978 (2014).
Hamatani, H. et al. TGF-β1 alters DNA methylation levels in promoter and enhancer regions of the WT1 gene in human podocytes. Nephrology 24, 575–584 (2019).
Zhou, L. et al. Mutual antagonism of Wilms’ tumor 1 and β-catenin dictates podocyte health and disease. J. Am. Soc. Nephrol. 26, 677–691 (2015).
Dehbi, M., Hiscott, J. & Pelletier, J. Activation of the wt1 Wilms’ tumor suppressor gene by NF-κB. Oncogene 16, 2033–2039 (1998).
Arellano-Rodríguez, M. et al. The inflammatory process modulates the expression and localization of WT1 in podocytes leading to kidney damage. In Vivo 35, 3137–3146 (2021).
Wang, D., Li, Y., Wu, C. & Liu, Y. PINCH1 is transcriptional regulator in podocytes that interacts with WT1 and represses podocalyxin expression. PLoS ONE 6, e17048 (2011).
Zhang, S.-Y. et al. CMIP interacts with WT1 and targets it on the proteasome degradation pathway. Clin. Transl. Med. 11, e460 (2021).
Rico, M. et al. WT1-interacting protein and ZO-1 translocate into podocyte nuclei after puromycin aminonucleoside treatment. Am. J. Physiol. Renal Physiol. 289, F431–F441 (2005).
Gebeshuber, C. A. et al. Focal segmental glomerulosclerosis is induced by microRNA-193a and its downregulation of WT1. Nat. Med. 19, 481–487 (2013).
Wang, Q., Ge, X., Zhang, J. & Chen, L. Effect of lncRNA WT1-AS regulating WT1 on oxidative stress injury and apoptosis of neurons in Alzheimer’s disease via inhibition of the miR-375/SIX4 axis. Aging 12, 23974–23995 (2020).
Sharma, P. M., Bowman, M., Madden, S. L., Rauscher, F. J. III & Sukumar, S. RNA editing in the Wilms’ tumor susceptibility gene, WT1. Genes. Dev. 8, 720–731 (1994).
Kolch, W., Halasz, M., Granovskaya, M. & Kholodenko, B. N. The dynamic control of signal transduction networks in cancer cells. Nat. Rev. Cancer 15, 515–527 (2015).
Liu, G. Y. & Sabatini, D. M. mTOR at the nexus of nutrition, growth, ageing and disease. Nat. Rev. Mol. Cell Biol. 21, 183–203 (2020).
Huynh, C., Ryu, J., Lee, J., Inoki, A. & Inoki, K. Nutrient-sensing mTORC1 and AMPK pathways in chronic kidney diseases. Nat. Rev. Nephrol. 19, 102–122 (2023).
Koehler, F. C., Späth, M. R., Hoyer-Allo, K. J. R. & Müller, R.-U. Mechanisms of caloric restriction-mediated stress-resistance in acute kidney injury. Nephron 146, 234–238 (2022).
Mitchell, J. R. et al. Short-term dietary restriction and fasting precondition against ischemia reperfusion injury in mice. Aging Cell 9, 40–53 (2010).
Späth, M. R. et al. Preconditioning strategies to prevent acute kidney injury. F1000Res. 9, 237 (2020).
Xie, R. et al. Identifying progression related disease risk modules based on the human subcellular signaling networks. Mol. Biosyst. 10, 3298–3309 (2014).
Irish, J. M. et al. B-cell signaling networks reveal a negative prognostic human lymphoma cell subset that emerges during tumor progression. Proc. Natl Acad. Sci. USA 107, 12747–12754 (2010).
Tognetti, M. et al. Deciphering the signaling network of breast cancer improves drug sensitivity prediction. Cell Syst. 12, 401–418.e12 (2021).
Saez-Rodriguez, J. & Blüthgen, N. Personalized signaling models for personalized treatments. Mol. Syst. Biol. 16, e9042 (2020).
Kanehisa, M., Furumichi, M., Sato, Y., Kawashima, M. & Ishiguro-Watanabe, M. KEGG for taxonomy-based analysis of pathways and genomes. Nucleic Acids Res. 51, D587–D592 (2023).
Gillespie, M. et al. The reactome pathway knowledgebase 2022. Nucleic Acids Res. 50, D687–D692 (2022).
Garcia-Alonso, L. et al. Single-cell roadmap of human gonadal development. Nature 607, 540–547 (2022).
Jin, S. et al. Inference and analysis of cell-cell communication using CellChat. Nat. Commun. 12, 1088 (2021).
Türei, D. et al. Integrated intra- and intercellular signaling knowledge for multicellular omics analysis. Mol. Syst. Biol. 17, e9923 (2021).
Garrido-Rodriguez, M., Zirngibl, K., Ivanova, O., Lobentanzer, S. & Saez-Rodriguez, J. Integrating knowledge and omics to decipher mechanisms via large-scale models of signaling networks. Mol. Syst. Biol. 18, e11036 (2022).
Piehl, N. et al. Cerebrospinal fluid immune dysregulation during healthy brain aging and cognitive impairment. Cell 185, 5028–5039.e13 (2022).
Billmann, M., Chaudhary, V., ElMaghraby, M. F., Fischer, B. & Boutros, M. Widespread rewiring of genetic networks upon cancer signaling pathway activation. Cell Syst. 6, 52–64.e4 (2018).
Sanchez-Vega, F. et al. Oncogenic signaling pathways in the cancer genome atlas. Cell 173, 321–337.e10 (2018).
Downward, J. Targeting RAS signalling pathways in cancer therapy. Nat. Rev. Cancer 3, 11–22 (2003).
Schubbert, S., Shannon, K. & Bollag, G. Hyperactive Ras in developmental disorders and cancer. Nat. Rev. Cancer 7, 295–308 (2007).
Menezes, L. F. & Germino, G. G. The pathobiology of polycystic kidney disease from a metabolic viewpoint. Nat. Rev. Nephrol. 15, 735–749 (2019).
Zhou, X. & Torres, V. E. Emerging therapies for autosomal dominant polycystic kidney disease with a focus on cAMP signaling. Front. Mol. Biosci. 9, 981963 (2022).
Baghdadi, M., Hinterding, H. M., Partridge, L. & Deelen, J. From mutation to mechanism: deciphering the molecular function of genetic variants linked to human ageing. Brief. Funct. Genomics 21, 13–23 (2022).
Fraser, H. C. et al. Biological mechanisms of aging predict age-related disease co-occurrence in patients. Aging Cell 21, e13524 (2022).
Gallagher, M. D. & Chen-Plotkin, A. S. The post-GWAS era: from association to function. Am. J. Hum. Genet. 102, 717–730 (2018).
Johnson, J. R. et al. Global post-translational modification profiling of HIV-1-infected cells reveals mechanisms of host cellular pathway remodeling. Cell Rep. 39, 110690 (2022).
Skowronek, P. et al. Rapid and in-depth coverage of the (phospho-)proteome with deep libraries and optimal window design for dia-PASEF. Mol. Cell. Proteom. 21, 100279 (2022).
Rinschen, M. M. & Saez-Rodriguez, J. The tissue proteome in the multi-omic landscape of kidney disease. Nat. Rev. Nephrol. 17, 205–219 (2021).
Gatto, L. et al. Initial recommendations for performing, benchmarking and reporting single-cell proteomics experiments. Nat. Methods 20, 375–386 (2023).
Szalai, B. & Saez-Rodriguez, J. Why do pathway methods work better than they should? FEBS Lett. 594, 4189–4200 (2020).
Birrell, G. W. et al. Transcriptional response of Saccharomyces cerevisiae to DNA-damaging agents does not identify the genes that protect against these agents. Proc. Natl Acad. Sci. USA 99, 8778–8783 (2002).
Begley, T. J., Rosenbach, A. S., Ideker, T. & Samson, L. D. Damage recovery pathways in Saccharomyces cerevisiae revealed by genomic phenotyping and interactome mapping. Mol. Cancer Res. 1, 103–112 (2002).
Workman, C. T. et al. A systems approach to mapping DNA damage response pathways. Science 312, 1054–1059 (2006).
Schacht, T., Oswald, M., Eils, R., Eichmüller, S. B. & König, R. Estimating the activity of transcription factors by the effect on their target genes. Bioinformatics 30, i401–i407 (2014).
Lee, E. & Bussemaker, H. J. Identifying the genetic determinants of transcription factor activity. Mol. Syst. Biol. 6, 412 (2010).
Yang, J. et al. Super-enhancer-associated transcription factors maintain transcriptional regulation in mature podocytes. J. Am. Soc. Nephrol. 32, 1323–1337 (2021).
Lackner, A. et al. Cooperative genetic networks drive embryonic stem cell transition from naïve to formative pluripotency. EMBO J. 40, e105776 (2021).
Garcia-Alonso, L., Holland, C. H., Ibrahim, M. M., Turei, D. & Saez-Rodriguez, J. Benchmark and integration of resources for the estimation of human transcription factor activities. Genome Res. 29, 1363–1375 (2019).
Keenan, A. B. et al. ChEA3: transcription factor enrichment analysis by orthogonal omics integration. Nucleic Acids Res. 47, W212–W224 (2019).
Liu, Z.-P., Wu, C., Miao, H. & Wu, H. RegNetwork: an integrated database of transcriptional and post-transcriptional regulatory networks in human and mouse. Database 2015, bav095 (2015).
Schubert, M. et al. Perturbation-response genes reveal signaling footprints in cancer gene expression. Nat. Commun. 9, 20 (2018).
Holland, C. H. et al. Robustness and applicability of transcription factor and pathway analysis tools on single-cell RNA-seq data. Genome Biol. 21, 36 (2020).
Kuppe, C. et al. Decoding myofibroblast origins in human kidney fibrosis. Nature 589, 281–286 (2021).
Weidemüller, P., Kholmatov, M., Petsalaki, E. & Zaugg, J. B. Transcription factors: bridge between cell signaling and gene regulation. Proteomics 21, e2000034 (2021).
Wilkinson, A. C., Nakauchi, H. & Göttgens, B. Mammalian transcription factor networks: recent advances in interrogating biological complexity. Cell Syst. 5, 319–331 (2017).
Kann, M. et al. WT1 targets Gas1 to maintain nephron progenitor cells by modulating FGF signals. Development 142, 1254–1266 (2015).
Neph, S. et al. Circuitry and dynamics of human transcription factor regulatory networks. Cell 150, 1274–1286 (2012).
Bar-Joseph, Z. et al. Computational discovery of gene modules and regulatory networks. Nat. Biotechnol. 21, 1337–1342 (2003).
Badia-I-Mompel, P. et al. Gene regulatory network inference in the era of single-cell multi-omics. Nat. Rev. Genet. 24, 739–754 (2023).
Banerji, J., Rusconi, S. & Schaffner, W. Expression of a β-globin gene is enhanced by remote SV40 DNA sequences. Cell 27, 299–308 (1981).
Fulco, C. P. et al. Activity-by-contact model of enhancer-promoter regulation from thousands of CRISPR perturbations. Nat. Genet. 51, 1664–1669 (2019).
Allocco, D. J., Kohane, I. S. & Butte, A. J. Quantifying the relationship between co-expression, co-regulation and gene function. BMC Bioinforma. 5, 18 (2004).
Gao, F., Foat, B. C. & Bussemaker, H. J. Defining transcriptional networks through integrative modeling of mRNA expression and transcription factor binding data. BMC Bioinforma. 5, 31 (2004).
Bulyk, M. L. Computational prediction of transcription-factor binding site locations. Genome Biol. 5, 201 (2003).
Beyer, A. et al. Integrated assessment and prediction of transcription factor binding. PLoS Comput. Biol. 2, e70 (2006).
Buenrostro, J. D. et al. Single-cell chromatin accessibility reveals principles of regulatory variation. Nature 523, 486–490 (2015).
Taverner, N. V., Smith, J. C. & Wardle, F. C. Identifying transcriptional targets. Genome Biol. 5, 210 (2004).
Redestig, H., Weicht, D., Selbig, J. & Hannah, M. A. Transcription factor target prediction using multiple short expression time series from Arabidopsis thaliana. BMC Bioinforma. 8, 454 (2007).
Gupta, A. et al. Inferring gene regulation from stochastic transcriptional variation across single cells at steady state. Proc. Natl Acad. Sci. USA 119, e2207392119 (2022).
He, B. & Tan, K. Understanding transcriptional regulatory networks using computational models. Curr. Opin. Genet. Dev. 37, 101–108 (2016).
Duren, Z., Chen, X., Jiang, R., Wang, Y. & Wong, W. H. Modeling gene regulation from paired expression and chromatin accessibility data. Proc. Natl Acad. Sci. USA 114, E4914–E4923 (2017).
Kleino, I., Frolovaitė, P., Suomi, T. & Elo, L. L. Computational solutions for spatial transcriptomics. Comput. Struct. Biotechnol. J. 20, 4870–4884 (2022).
Wagner, J. et al. Medin co-aggregates with vascular amyloid-β in Alzheimer’s disease. Nature 612, 123–131 (2022).
Ramachandran, P. et al. Resolving the fibrotic niche of human liver cirrhosis at single-cell level. Nature 575, 512–518 (2019).
Liu, S. et al. Single-cell transcriptomics reveals a mechanosensitive injury signaling pathway in early diabetic nephropathy. Genome Med. 15, 2 (2023).
Balzer, M. S. et al. Single-cell analysis highlights differences in druggable pathways underlying adaptive or fibrotic kidney regeneration. Nat. Commun. 13, 4018 (2022).
Duren, Z. et al. Regulatory analysis of single cell multiome gene expression and chromatin accessibility data with scREG. Genome Biol. 23, 114 (2022).
Bravo González-Blas, C. et al. SCENIC+: single-cell multiomic inference of enhancers and gene regulatory networks. Nat. Methods 20, 1355–1367 (2023).
Muto, Y. et al. Single cell transcriptional and chromatin accessibility profiling redefine cellular heterogeneity in the adult human kidney. Nat. Commun. 12, 2190 (2021).
Miao, Z. et al. Single cell regulatory landscape of the mouse kidney highlights cellular differentiation programs and disease targets. Nat. Commun. 12, 2277 (2021).
O’Brown, Z. K., Van Nostrand, E. L., Higgins, J. P. & Kim, S. K. The inflammatory transcription factors NFκB, STAT1 and STAT3 drive age-associated transcriptional changes in the human kidney. PLoS Genet. 11, e1005734 (2015).
van Dam, S., Võsa, U., van der Graaf, A., Franke, L. & de Magalhães, J. P. Gene co-expression analysis for functional classification and gene-disease predictions. Brief. Bioinform. 19, 575–592 (2018).
Deelen, P. et al. Improving the diagnostic yield of exome-sequencing by predicting gene–phenotype associations using large-scale gene expression analysis. Nat. Commun. 10, 2837 (2019).
Leote, A. C., Wu, X. & Beyer, A. Regulatory network-based imputation of dropouts in single-cell RNA sequencing data. PLoS Comput. Biol. 18, e1009849 (2022).
Lee, T. I. et al. Transcriptional regulatory networks in Saccharomyces cerevisiae. Science 298, 799–804 (2002).
van Roeyen, C. R. C. et al. Growth arrest-specific protein 1 is a novel endogenous inhibitor of glomerular cell activation and proliferation. Kidney Int. 83, 251–263 (2013).
Gennarino, V. A. et al. MicroRNA target prediction by expression analysis of host genes. Genome Res. 19, 481–490 (2009).
Marco, A., Konikoff, C., Karr, T. L. & Kumar, S. Relationship between gene co-expression and sharing of transcription factor binding sites in Drosophila melanogaster. Bioinformatics 25, 2473–2477 (2009).
Hinnebusch, A. G. & Natarajan, K. Gcn4p, a master regulator of gene expression, is controlled at multiple levels by diverse signals of starvation and stress. Eukaryot. Cell 1, 22–32 (2002).
Komili, S. & Silver, P. A. Coupling and coordination in gene expression processes: a systems biology view. Nat. Rev. Genet. 9, 38–48 (2008).
Liu, Y., Beyer, A. & Aebersold, R. On the dependency of cellular protein levels on mRNA abundance. Cell 165, 535–550 (2016).
Emmert-Streib, F. Influence of the experimental design of gene expression studies on the inference of gene regulatory networks: environmental factors. PeerJ 1, e10 (2013).
Gross, T. & Blüthgen, N. Identifiability and experimental design in perturbation studies. Bioinformatics 36, i482–i489 (2020).
Mombaerts, L., Aalto, A., Markdahl, J. & Gonçalves, J. A multifactorial evaluation framework for gene regulatory network reconstruction. IFAC-PapersOnLine 52, 262–268 (2019).
Liu, S. et al. Weighted gene co-expression network analysis identifies FCER1G as a key gene associated with diabetic kidney disease. Ann. Transl. Med. 8, 1427 (2020).
Osuna-Martinez, U. et al. In silico prediction of hub genes involved in diabetic kidney and COVID-19 related disease by differential gene expression and interactome analysis. Genes 13, 2412 (2022).
Harris, B. D., Crow, M., Fischer, S. & Gillis, J. Single-cell co-expression analysis reveals that transcriptional modules are shared across cell types in the brain. Cell Syst. 12, 748–756.e3 (2021).
Zhang, J. & Zhang, S. in Encyclopedia of Systems Biology (eds Dubitzky, W., Wolkenhauer, O., Cho, K.-H. & Yokota, H.) 1437–1441 (Springer New York, 2013).
Møller, A. F. & Natarajan, K. N. Predicting gene regulatory networks from cell atlases. Life Sci. Alliance 3, e202000658 (2020).
Cleary, B., Cong, L., Cheung, A., Lander, E. S. & Regev, A. Efficient generation of transcriptomic profiles by random composite measurements. Cell 171, 1424–1436.e18 (2017).
Scarpulla, R. C., Vega, R. B. & Kelly, D. P. Transcriptional integration of mitochondrial biogenesis. Trends Endocrinol. Metab. 23, 459–466 (2012).
Weith, M. et al. Genetic effects on molecular network states explain complex traits. Mol. Syst. Biol. 19, e11493 (2023).
Suo, S. et al. Revealing the critical regulators of cell identity in the mouse cell atlas. Cell Rep. 25, 1436–1445.e3 (2018).
Lotfollahi, M. et al. Predicting cellular responses to complex perturbations in high-throughput screens. Mol. Syst. Biol. 19, e11517 (2023).
Roohani, Y., Huang, K. & Leskovec, J. Predicting transcriptional outcomes of novel multigene perturbations with GEARS. Nat. Biotechnol. https://doi.org/10.1038/s41587-023-01905-6 (2023).
Boulogne, F. et al. KidneyNetwork: using kidney-derived gene expression data to predict and prioritize novel genes involved in kidney disease. Eur. J. Hum. Genet. 31, 1300–1308 (2023).
Mignone, P., Pio, G., D’Elia, D. & Ceci, M. Exploiting transfer learning for the reconstruction of the human gene regulatory network. Bioinformatics 36, 1553–1561 (2020).
Kuijjer, M. L., Tung, M. G., Yuan, G., Quackenbush, J. & Glass, K. Estimating sample-specific regulatory networks. iScience 14, 226–240 (2019).
Coleman, L. A. et al. Personal network inference unveils heterogeneous immune response patterns to viral infection in children with acute wheezing. J. Pers. Med. 11, 1293 (2021).
Reznik, E. & Sander, C. Extensive decoupling of metabolic genes in cancer. PLoS Comput. Biol. 11, e1004176 (2015).
Kostka, D. & Spang, R. Finding disease specific alterations in the co-expression of genes. Bioinformatics 20, i194–i199 (2004).
de la Fuente, A. From ‘differential expression’ to ‘differential networking’ — identification of dysfunctional regulatory networks in diseases. Trends Genet. 26, 326–333 (2010).
Truong, T. T. et al. Co-expression networks unveiled long non-coding RNAs as molecular targets of drugs used to treat bipolar disorder. Front. Pharmacol. 13, 873271 (2022).
Moerman, T. et al. GRNBoost2 and Arboreto: efficient and scalable inference of gene regulatory networks. Bioinformatics 35, 2159–2161 (2019).
López-Otín, C., Blasco, M. A., Partridge, L., Serrano, M. & Kroemer, G. Hallmarks of aging: an expanding universe. Cell 186, 243–278 (2023).
Fritsch, C. et al. Genome-wide surveillance of transcription errors in response to genotoxic stress. Proc. Natl Acad. Sci. USA 118, e2004077118 (2021).
Debès, C. et al. Ageing-associated changes in transcriptional elongation influence longevity. Nature 616, 814–821 (2023).
Heintz, C. et al. Splicing factor 1 modulates dietary restriction and TORC1 pathway longevity in C. elegans. Nature 541, 102–106 (2017).
Bahar, R. et al. Increased cell-to-cell variation in gene expression in ageing mouse heart. Nature 441, 1011–1014 (2006).
Gyenis, A. et al. Genome-wide RNA polymerase stalling shapes the transcriptome during aging. Nat. Genet. 55, 268–279 (2023).
Papadakis, A. et al. Age-associated transcriptional stress due to accelerated elongation and increased stalling of RNAPII. Nat. Genet. 55, 2011–2012 (2023).
Elowitz, M. B., Levine, A. J., Siggia, E. D. & Swain, P. S. Stochastic gene expression in a single cell. Science 297, 1183–1186 (2002).
Angelidis, I. et al. An atlas of the aging lung mapped by single cell transcriptomics and deep tissue proteomics. Nat. Commun. 10, 1–17 (2019).
Ibañez-Solé, O., Ascensión, A. M., Araúzo-Bravo, M. J. & Izeta, A. Lack of evidence for increased transcriptional noise in aged tissues. Elife 11, e80380 (2022).
Lake, B. B. et al. An atlas of healthy and injured cell states and niches in the human kidney. Nature 619, 585–594 (2023).
Bravo González-Blas, C. et al. Identification of genomic enhancers through spatial integration of single-cell transcriptomics and epigenomics. Mol. Syst. Biol. 16, e9438 (2020).
Williams, G. C. Pleiotropy, natural selection, and the evolution of senescence. Evolution 11, 398 (1957).
Bochdanovits, Z. & de Jong, G. Antagonistic pleiotropy for life-history traits at the gene expression level. Proc. Biol. Sci. 271, S75–S78 (2004).
Long, E. & Zhang, J. Evidence for the role of selection for reproductively advantageous alleles in human aging. Sci. Adv. 9, eadh4990 (2023).
Zhang, J. Patterns and evolutionary consequences of pleiotropy. Annu. Rev. Ecol. Evol. Syst. 54, 1–19 (2023).
Huynh-Thu, V. A. & Sanguinetti, G. in Gene Regulatory Networks: Methods and Protocols (eds Sanguinetti, G. & Huynh-Thu, V. A.) 1–23 (Springer New York, 2019).
Beyer, A., Bandyopadhyay, S. & Ideker, T. Integrating physical and genetic maps: from genomes to interaction networks. Nat. Rev. Genet. 8, 699–710 (2007).
Langfelder, P. & Horvath, S. WGCNA: an R package for weighted correlation network analysis. BMC Bioinforma. 9, 559 (2008).
Margolin, A. A. et al. ARACNE: an algorithm for the reconstruction of gene regulatory networks in a mammalian cellular context. BMC Bioinforma. 7, S7 (2006).
Meyer, P. E., Kontos, K., Lafitte, F. & Bontempi, G. Information-theoretic inference of large transcriptional regulatory networks. EURASIP J. Bioinform. Syst. Biol. 2007, 79879 (2007).
Haury, A.-C., Mordelet, F., Vera-Licona, P. & Vert, J.-P. TIGRESS: trustful inference of gene regulation using stability selection. BMC Syst. Biol. 6, 145 (2012).
Hill, S. M. et al. Inferring causal molecular networks: empirical assessment through a community-based effort. Nat. Methods 13, 310–318 (2016).
Huynh-Thu, V. A., Irrthum, A., Wehenkel, L. & Geurts, P. Inferring regulatory networks from expression data using tree-based methods. PLoS ONE 5, e12776 (2010).
Friedman, N., Linial, M., Nachman, I. & Pe’er, D. Using Bayesian networks to analyze expression data. J. Comput. Biol. 7, 601–620 (2000).
Huntington-Klein, N. The Effect: An Introduction to Research Design and Causality (Chapman and Hall/CRC Press, 2021).
Pearl, J. Causality (Cambridge Univ. Press, 2009).
Kalisch, M., Mächler, M., Colombo, D., Maathuis, M. H. & Bühlmann, P. Causal inference using graphical models with the R package pcalg. J. Stat. Softw. 47, 1–26 (2012).
Squires, C. & Uhler, C. Causal structure learning: a combinatorial perspective. Found. Comut. Math. 1, 1–35 (2022).
Heinze-Deml, C., Maathuis, M. H. & Meinshausen, N. Causal structure learning. Annu. Rev. Stat. Appl. 5, 371–391 (2018).
Triantafillou, S. et al. Predicting causal relationships from biological data: applying automated causal discovery on mass cytometry data of human immune cells. Sci. Rep. 7, 12724 (2017).
Hu, H., Li, Z. & Vetta, A. R. in Advances in Neural Information Processing Systems (eds Ghahramani, Z., Welling, M., Cortes, C., Lawrence, N. & Weinberger, K. Q.). 27 (Curran Associates, 2014).
Auwerx, C. et al. Exploiting the mediating role of the metabolome to unravel transcript-to-phenotype associations. Elife 12, e81097 (2023).
Shojaie, A. & Michailidis, G. Discovering graphical Granger causality using the truncating lasso penalty. Bioinformatics 26, i517–i523 (2010).
Hill, S. M. et al. Context specificity in causal signaling networks revealed by phosphoprotein profiling. Cell Syst. 4, 73–83.e10 (2017).
Lu, J. et al. Causal network inference from gene transcriptional time-series response to glucocorticoids. PLoS Comput. Biol. 17, e1008223 (2021).
Aalto, A., Viitasaari, L., Ilmonen, P., Mombaerts, L. & Gonçalves, J. Gene regulatory network inference from sparsely sampled noisy data. Nat. Commun. 11, 3493 (2020).
Meinshausen, N. et al. Methods for causal inference from gene perturbation experiments and validation. Proc. Natl Acad. Sci. USA 113, 7361–7368 (2016).
Dixit, A. et al. Perturb-seq: dissecting molecular circuits with scalable single-cell RNA profiling of pooled genetic screens. Cell 167, 1853–1866.e17 (2016).
Liu, B., de la Fuente, A. & Hoeschele, I. Gene network inference via structural equation modeling in genetical genomics experiments. Genetics 178, 1763–1776 (2008).
Aygün, N. et al. Inferring cell-type-specific causal gene regulatory networks during human neurogenesis. Genome Biol. 24, 130 (2023).
Lecca, P. Machine learning for causal inference in biological networks: perspectives of this challenge. Front. Bioinform 1, 746712 (2021).
- The Renal Warrior Project. Join Now
- Source: https://www.nature.com/articles/s41581-024-00849-7